Artificial Intelligence (AI) has become a game-changer in various industries, including cybersecurity. Insider threats, defined as threats that come from within an organization, pose a considerable risk to businesses. In response to this, many companies have turned to AI to detect and prevent such threats. However, implementing AI for insider threat detection is not without its challenges. In this essay, we will explore some of the key challenges in implementing AI for insider threat detection.
The first challenge is data quality. AI algorithms require high-quality data to perform effectively. In the case of insider threat detection, this means that data must be collected from multiple sources, including email logs, chat logs, network logs, and user activity logs. This data must be accurate, complete, and up-to-date. Unfortunately, collecting and organizing this data is a complex and time-consuming task. Furthermore, it is not uncommon for data to be incomplete or inaccurate, which can lead to false positives or false negatives. Therefore, organizations need to invest in data cleaning and preprocessing tools to ensure that the data is accurate and reliable.
The second challenge is data privacy. Insider threat detection requires access to sensitive data, including personal information and confidential company data. However, accessing this data can violate privacy laws and regulations, such as the European Union's General Data Protection Regulation (GDPR) or the California Consumer Privacy Act (CCPA). Therefore, organizations must take steps to ensure that the data they collect is handled in compliance with these regulations. This may involve anonymizing the data, restricting access to authorized personnel, or obtaining explicit consent from employees.
The third challenge is algorithm accuracy. AI algorithms are only as good as the data they are trained on. In the case of insider threat detection, the algorithms must be trained on a diverse set of data that includes both normal and abnormal user behavior. Unfortunately, identifying abnormal behavior can be challenging, as it may not always be clear what constitutes normal behavior. Therefore, organizations need to invest in high-quality training data and use advanced machine learning techniques to identify anomalous behavior accurately.
The fourth challenge is model explainability. AI algorithms can be difficult to understand and explain, which can make it challenging to identify and address false positives or false negatives. In the case of insider threat detection, it is essential to understand why an algorithm has flagged a particular user as a potential threat. Therefore, organizations need to invest in tools that can explain the reasoning behind AI decisions.
The fifth challenge is human error. AI algorithms are not infallible and can make mistakes. Therefore, it is essential to have human oversight of the algorithm and to ensure that the results are verified before taking action. Furthermore, employees may deliberately or accidentally try to evade detection by the AI system. Therefore, organizations need to provide training to employees to ensure that they understand the importance of cybersecurity and to monitor employee behavior for signs of malfeasance. In conclusion, implementing AI for insider threat detection presents several challenges, including data quality, data privacy, algorithm accuracy, model explainability, and human error. Organizations must invest in the right tools and technologies to overcome these challenges and ensure that their AI systems are effective in detecting and preventing insider threats. With the right approach, AI can be a powerful tool for improving cybersecurity and protecting organizations from the risks posed by insider threats.
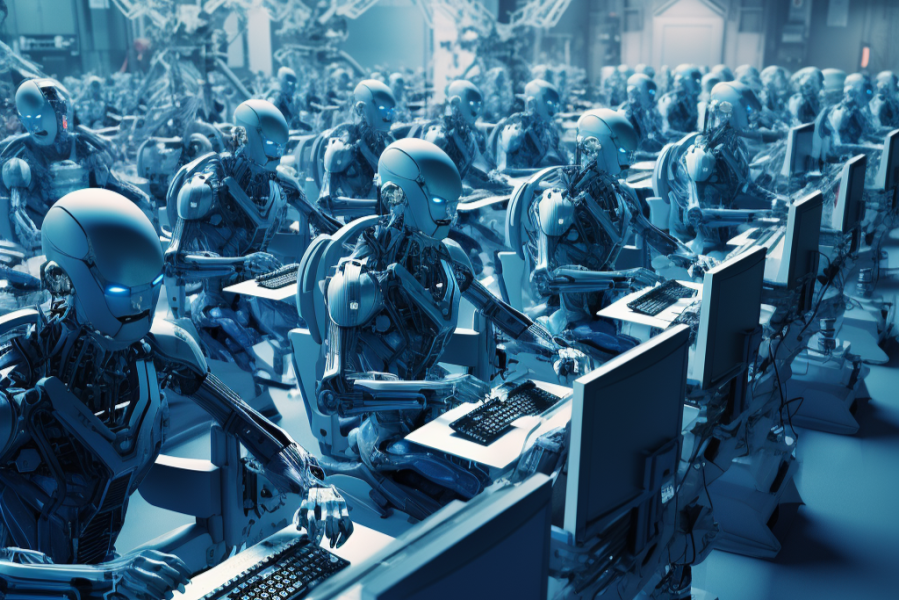
Strategic Advisor, ShadowSight
Who is Christopher McNaughton
Chris is a proficient problem solver with a strategic aptitude for anticipating and addressing potential business issues, particularly in areas such as Insider Threat, Data Governance, Digital Forensics, Workplace Investigations, and Cyber Security. He thrives on turning intricate challenges into opportunities for increased efficiency, offering pragmatic solutions derived from a practical and realistic approach.
Starting his career as a law enforcement Detective, Chris transitioned to multinational organisations where he specialised and excelled in Cyber Security, proving his authority in the field. Even under demanding circumstances, his commitment to delivering exceptional results remains unwavering, underpinned by his extraordinary ability to understand both cyber and business problems swiftly, along with a deep emphasis on active listening.
What is ShadowSight
ShadowSight is an innovative insider risk staff monitoring tool that proactively guards your business against internal threats and safeguards vital data from unauthorised access and malicious activities. We offer a seamless integration with your current systems, boosting regulatory compliance while providing unparalleled visibility into non-compliant activities to reinforce a secure digital environment. By prioritising actionable intelligence, ShadowSight not only mitigates insider threats but also fosters a culture of proactive risk management, significantly simplifying your compliance process without the overwhelming burden of false positives.